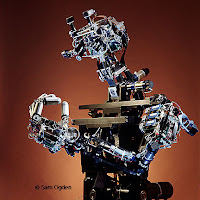
An expert system is software that attempts to provide an answer to a problem, or clarify uncertainties where normally one or more human experts would need to be consulted. Expert systems are most common in a specific problem domain, and is a traditional application and/or subfield of artificial intelligence. A wide variety of methods can be used to simulate the performance of the expert however common to most or all are 1) the creation of a so-called "knowledgebase" which uses some knowledge representation formalism to capture the Subject Matter Expert's (SME) knowledge and 2) a process of gathering that knowledge from the SME and codifying it according to the formalism, which is called knowledge engineering. Expert systems may or may not have learning components but a third common element is that once the system is developed it is proven by being placed in the same real world problem solving situation as the human SME, typically as an aid to human workers or a supplement to some information system.
Expert systems were introduced by Edward Feigenbaum, the first truly successful form of AI software. The topic of expert systems has many points of contact with general systems theory, operations research, business process reengineering and various topics in applied mathematics and management science.
Expert System Topics
--------------------
ChainingTwo methods of reasoning when using inference rules are backward chaining and forward chaining.
Forward chaining starts with the data available and uses the inference rules to conclude more data until a desired goal is reached. An inference engine using forward chaining searches the inference rules until it finds one in which the if clause is known to be true. It then concludes the then clause and adds this information to its data. It would continue to do this until a goal is reached. Because the data available determines which inference rules are used, this method is also called data driven.
Backward chaining starts with a list of goals and works backwards to see if there is data which will allow it to conclude any of these goals. An inference engine using backward chaining would search the inference rules until it finds one which has a then clause that matches a desired goal. If the if clause of that inference rule is not known to be true, then it is added to the list of goals. For example, suppose a rule base contains
1. If Fritz is green then Fritz is a frog.
2. If Fritz is a frog then Fritz hops.
Suppose a goal is to conclude that Fritz hops. The rule base would be searched and rule (2) would be selected because its conclusion (the then clause) matches the goal. It is not known that Fritz is a frog, so this "if" statement is added to the goal list. The rule base is again searched and this time rule (1) is selected because its then clause matches the new as certainty factors. A human, when reasoning, does not always conclude things with 100% confidence: he might venture, "If Fritz is green, then he is probably a frog" (after all, he might be a chameleon). This type of reasoning can be imitated by using numeric values called confidences. For example, if it is known that Fritz is green, it might be concluded with 0.85 confidence that he is a frog; or, if it is known that he is a frog, it might be concluded with 0.95 confidence that he hops. These numbers are probabilities in a Bayesian sense, in that they quantify uncertainty.
Expert system architectureThe following general points about expert systems and their architecture have been illustrated.
1. The sequence of steps taken to reach a conclusion is dynamically synthesized with each new case. It is not explicitly programmed when the system is built.
2. Expert systems can process multiple values for any problem parameter. This permits more than one line of reasoning to be pursued and the results of incomplete (not fully determined) reasoning to be presented.
3. Problem solving is accomplished by applying specific knowledge rather than specific technique. This is a key idea in expert systems technology. It reflects the belief that human experts do not process their knowledge differently from others, but they do possess different knowledge. With this philosophy, when one finds that their expert system does not produce the desired results, work begins to expand the knowledge base, not to re-program the procedures.
There are various expert systems in which a rulebase and an inference engine cooperate to simulate the reasoning process that a human expert pursues in analyzing a problem and arriving at a conclusion. In these systems, in order to simulate the human reasoning process, a vast amount of knowledge needed to be stored in the knowledge base. Generally, the knowledge base of such an expert system consisted of a relatively large number of "if then" type of statements that were interrelated in a manner that, in theory at least, resembled the sequence of mental steps that were involved in the human reasoning process.
Because of the need for large storage capacities and related programs to store the rulebase, most expert systems have, in the past, been run only on large information handling systems. Recently, the storage capacity of personal computers has increased to a point where it is becoming possible to consider running some types of simple expert systems on personal computers.
In some applications of expert systems, the nature of the application and the amount of stored information necessary to simulate the human reasoning process for that application is just too vast to store in the active memory of a computer. In other applications of expert systems, the nature of the application is such that not all of the information is always needed in the reasoning process. An example of this latter type application would be the use of an expert system to diagnose a data processing system comprising many separate components, some of which are optional. When that type of expert system employs a single integrated rulebase to diagnose the minimum system configuration of the data processing system, much of the rulebase is not required since many of the components which are optional units of the system will not be present in the system. Nevertheless, earlier expert systems require the entire rulebase to be stored since all the rules were, in effect, chained or linked together by the structure of the rulebase.
When the rulebase is segmented, preferably into contextual segments or units, it is then possible to eliminate portions of the Rulebase containing data or knowledge that is not needed in a particular application. The segmenting of the rulebase also allows the expert system to be run with systems or on systems having much smaller memory capacities than was possible with earlier arrangements since each segment of the rulebase can be paged into and out of the system as needed. The segmenting of the rulebase into contextual segments requires that the expert system manage various intersegment relationships as segments are paged into and out of memory during execution of the program. Since the system permits a rulebase segment to be called and executed at any time during the processing of the first rulebase, provision must be made to store the data that has been accumulated up to that point so that at some time later in the process, when the system returns to the first segment, it can proceed from the last point or rule node that was processed. Also, provision must be made so that data that has been collected by the system up to that point can be passed to the second segment of the rulebase after it has been paged into the system and data collected during the processing of the second segment can be passed to the first segment when the system returns to complete processing that segment.
The user interface and the procedure interface are two important functions in the information collection process.
End userThe end-user usually sees an expert system through an interactive dialog, an example of which follows:
Q. Do you know which restaurant you want to go to?
A. No
Q. Is there any kind of food you would particularly like?
A. No
Q. Do you like spicy food?
A. No
Q. Do you usually drink wine with meals?
A. Yes
Q. When you drink wine, is it French wine?
A. Yes
As can be seen from this dialog, the system is leading the user through a set of questions, the purpose of which is to determine a suitable set of restaurants to recommend. This dialog begins with the system asking if the user already knows the restaurant choice (a common feature of expert systems) and immediately illustrates a characteristic of expert systems; users may choose not to respond to any question. In expert systems, dialogs are not pre-planned. There is no fixed control structure. Dialogs are synthesized from the current information and the contents of the knowledge base. Because of this, not being able to supply the answer to a particular question does not stop the consultation.
Explanation systemAnother major distinction between expert systems and traditional systems is illustrated by the following answer given by the system when the user answers a question with another question, "Why", as occurred in the above example. The answer is:
A. I am trying to determine the type of restaurant to suggest. So far Chinese is not a likely choice. It is possible that French is a likely choice. I know that if the diner is a wine drinker, and the preferred wine is French, then there is strong evidence that the restaurant choice should include French.
It is very difficult to implement a general explanation system (answering questions like "Why" and "How") in a traditional computer program. An expert system can generate an explanation by retracing the steps of its reasoning. The response of the expert system to the question WHY is an exposure of the underlying knowledge structure. It is a rule; a set of antecedent conditions which, if true, allow the assertion of a consequent. The rule references values, and tests them against various constraints or asserts constraints onto them. This, in fact, is a significant part of the knowledge structure. There are values, which may be associated with some organizing entity. For example, the individual diner is an entity with various attributes (values) including whether they drink wine and the kind of wine. There are also rules, which associate the currently known values of some attributes with assertions that can be made about other attributes. It is the orderly processing of these rules that dictates the dialog itself.
Expert systems versus problem-solving systemsThe principal distinction between expert systems and traditional problem solving programs is the way in which the problem related expertise is coded. In traditional applications, problem expertise is encoded in both program and data structures. In the expert system approach all of the problem related expertise is encoded in data structures only; no problem-specific information is encoded in the program structure. This organization has several benefits.
An example may help contrast the traditional problem solving program with the expert system approach. The example is the problem of tax advice. In the traditional approach data structures describe the taxpayer and tax tables, and a program in which there are statements representing an expert tax consultant's knowledge, such as statements which relate information about the taxpayer to tax table choices. It is this representation of the tax expert's knowledge that is difficult for the tax expert to understand or modify.
In the expert system approach, the information about taxpayers and tax computations is again found in data structures, but now the knowledge describing the relationships between them is encoded in data structures as well. The programs of an expert system are independent of the problem domain (taxes) and serve to process the data structures without regard to the nature of the problem area they describe. For example, there are programs to acquire the described data values through user interaction, programs to represent and process special organizations of description, and programs to process the declarations that represent semantic relationships within the problem domain and an algorithm to control the processing sequence and focus.
The general architecture of an expert system involves two principal components: a problem dependent set of data declarations called the knowledge base or rule base, and a problem independent (although highly data structure dependent) program which is called the inference engine.
Individuals involved with expert systemsThere are generally three individuals having an interaction with expert systems. Primary among these is the end-user; the individual who uses the system for its problem solving assistance. In the building and maintenance of the system there are two other roles: the problem domain expert who builds and supplies the knowledge base providing the domain expertise, and a knowledge engineer who assists the experts in determining the representation of their knowledge, enters this knowledge into an explanation module and who defines the inference technique required to obtain useful problem solving activity. Usually, the knowledge engineer will represent the problem solving activity in the form of rules which is referred to as a rule-based expert system. When these rules are created from the domain expertise, the knowledge base stores the rules of the expert system.
Inference ruleAn understanding of the "inference rule" concept is important to understand expert systems. An inference rule is a statement that has two parts, an if clause and a then clause. This rule is what gives expert systems the ability to find solutions to diagnostic and prescriptive problems. An example of an inference rule is:
If the restaurant choice includes French, and the occasion is romantic,
Then the restaurant choice is definitely Paul Bocuse.
An expert system's rulebase is made up of many such inference rules. They are entered as separate rules and it is the inference engine that uses them together to draw conclusions. Because each rule is a unit, rules may be deleted or added without affecting other rules (though it should affect which conclusions are reached). One advantage of inference rules over traditional programming is that inference rules use reasoning which more closely resemble human reasoning.
Thus, when a conclusion is drawn, it is possible to understand how this conclusion was reached. Furthermore, because the expert system uses knowledge in a form similar to the expert, it may be easier to retrieve this information from the expert.
Procedure node interfaceThe function of the procedure node interface is to receive information from the procedures coordinator and create the appropriate procedure call. The ability to call a procedure and receive information from that procedure can be viewed as simply a generalization of input from the external world. While in some earlier expert systems external information has been obtained, that information was obtained only in a predetermined manner so only certain information could actually be acquired. This expert system, disclosed in the cross-referenced application, through the knowledge base, is permitted to invoke any procedure allowed on its host system. This makes the expert system useful in a much wider class of knowledge domains than if it had no external access or only limited external access.
In the area of machine diagnostics using expert systems, particularly self-diagnostic applications, it is not possible to conclude the current state of "health" of a machine without some information. The best source of information is the machine itself, for it contains much detailed information that could not reasonably be provided by the operator.
The knowledge that is represented in the system appears in the rulebase. In the rulebase described in the cross-referenced applications, there are basically four different types of objects, with associated information present.
1. Classes—these are questions asked to the user.
2. Parameters—a parameter is a place holder for a character string which may be a variable that can be inserted into a class question at the point in the question where the parameter is positioned.
3. Procedures—these are definitions of calls to external procedures.
4. Rule Nodes—The inferencing in the system is done by a tree structure which indicates the rules or logic which mimics human reasoning. The nodes of these trees are called rule nodes. There are several different types of rule nodes.
The rulebase comprises a forest of many trees. The top node of the tree is called the goal node, in that it contains the conclusion. Each tree in the forest has a different goal node. The leaves of the tree are also referred to as rule nodes, or one of the types of rule nodes. A leaf may be an evidence node, an external node, or a reference node.
An evidence node functions to obtain information from the operator by asking a specific question. In responding to a question presented by an evidence node, the operator is generally instructed to answer "yes" or "no" represented by numeric values 1 and 0 or provide a value of between 0 and 1, represented by a "maybe."
Questions which require a response from the operator other than yes or no or a value between 0 and 1 are handled in a different manner.
A leaf that is an external node indicates that data will be used which was obtained from a procedure call.
A reference node functions to refer to another tree or subtree.
A tree may also contain intermediate or minor nodes between the goal node and the leaf node. An intermediate node can represent logical operations like And or Or.
The inference logic has two functions. It selects a tree to trace and then it traces that tree. Once a tree has been selected, that tree is traced, depth-first, left to right.
The word "tracing" refers to the action the system takes as it traverses the tree, asking classes (questions), calling procedures, and calculating confidences as it proceeds.
As explained in the cross-referenced applications, the selection of a tree depends on the ordering of the trees. The original ordering of the trees is the order in which they appear in the rulebase. This order can be changed, however, by assigning an evidence node an attribute "initial" which is described in detail in these applications. The first action taken is to obtain values for all evidence nodes which have been assigned an "initial" attribute. Using only the answers to these initial evidences, the rules are ordered so that the most likely to succeed is evaluated first. The trees can be further re-ordered since they are constantly being updated as a selected tree is being traced.
It has been found that the type of information that is solicited by the system from the user by means of questions or classes should be tailored to the level of knowledge of the user. In many applications, the group of prospective uses is nicely defined and the knowledge level can be estimated so that the questions can be presented at a level which corresponds generally to the average user. However, in other applications, knowledge of the specific domain of the expert system might vary considerably among the group of prospective users.
One application where this is particularly true involves the use of an expert system, operating in a self-diagnostic mode on a personal computer to assist the operator of the personal computer to diagnose the cause of a fault or error in either the hardware or software. In general, asking the operator for information is the most straightforward way for the expert system to gather information assuming, of course, that the information is or should be within the operator's understanding. For example, in diagnosing a personal computer, the expert system must know the major functional components of the system. It could ask the operator, for instance, if the display is a monochrome or color display. The operator should, in all probability, be able to provide the correct answer 100% of the time. The expert system could, on the other hand, cause a test unit to be run to determine the type of display. The accuracy of the data collected by either approach in this instance probably would not be that different so the knowledge engineer could employ either approach without affecting the accuracy of the diagnosis. However, in many instances, because of the nature of the information being solicited, it is better to obtain the information from the system rather than asking the operator, because the accuracy of the data supplied by the operator is so low that the system could not effectively process it to a meaningful conclusion.
In many situations the information is already in the system, in a form of which permits the correct answer to a question to be obtained through a process of inductive or deductive reasoning. The data previously collected by the system could be answers provided by the user to less complex questions that were asked for a different reason or results returned from test units that were previously run.
User interfaceThe function of the user interface is to present questions and information to the user and supply the user's responses to the inference engine.
Any values entered by the user must be received and interpreted by the user interface. Some responses are restricted to a set of possible legal answers, others are not. The user interface checks all responses to insure that they are of the correct data type. Any responses that are restricted to a legal set of answers are compared against these legal answers. Whenever the user enters an illegal answer, the user interface informs the user that his answer was invalid and prompts him to correct it.
Application of expert systems
-----------------------------
Expert systems are designed and created to facilitate tasks in the fields of accounting, medicine, process control, financial service, production, human resources etc. Indeed, the foundation of a successful expert system depends on a series of technical procedures and development that may be designed by certain technicians and related experts.
A good example of application of expert systems in banking area is expert systems for mortgages. Loan departments are interested in expert systems for mortgages because of the growing cost of labour which makes the handling and acceptance of relatively small loans less profitable. They also see in the application of expert systems a possibility for standardised, efficient handling of mortgage loan, and appreciate that for the acceptance of mortgages there are hard and fast rules which do not always exist with other types of loans.
While expert systems have distinguished themselves in AI research in finding practical application, their application has been limited. Expert systems are notoriously narrow in their domain of knowledge—as an amusing example, a researcher used the "skin disease" expert system to diagnose his rustbucket car as likely to have developed measles—and the systems were thus prone to making errors that humans would easily spot. Additionally, once some of the mystique had worn off, most programmers realized that simple expert systems were essentially just slightly more elaborate versions of the decision logic they had already been using. Therefore, some of the techniques of expert systems can now be found in most complex programs without any fuss about them.
An example, and a good demonstration of the limitations of, an expert system used by many people is the Microsoft Windows operating system troubleshooting software located in the "help" section in the taskbar menu. Obtaining expert/technical operating system support is often difficult for individuals not closely involved with the development of the operating system. Microsoft has designed their expert system to provide solutions, advice, and suggestions to common errors encountered throughout using the operating systems.
Another 1970s and 1980s application of expert systems — which we today would simply call AI — was in computer games. For example, the computer baseball games Earl Weaver Baseball and Tony La Russa Baseball each had highly detailed simulations of the game strategies of those two baseball managers. When a human played the game against the computer, the computer queried the Earl Weaver or Tony La Russa Expert System for a decision on what strategy to follow. Even those choices where some randomness was part of the natural system (such as when to throw a surprise pitch-out to try to trick a runner trying to steal a base) were decided based on probabilities supplied by Weaver or La Russa. Today we would simply say that "the game's AI provided the opposing manager's strategy."
Advantages and disadvantagesAdvantages:
* Provides consistent answers for repetitive decisions, processes and tasks
* Holds and maintains significant levels of information
* Encourages organizations to clarify the logic of their decision-making
* Always asks a question, that a human might forget to ask
* Can work continuously (no human needs)
* Can be used by the user more frequently
* A multi-user expert system can serve more users at a time
Disadvantages:
* Lacks common sense needed in some decision making
* Cannot respond creatively like a human expert would in unusual circumstances
* Domain experts not always able to explain their logic and reasoning
* Errors may occur in the knowledge base, and lead to wrong decisions
* Cannot adapt to changing environments, unless knowledge base is changed
Types of problems solved by expert systemsExpert systems are most valuable to organizations that have a high-level of know-how experience and expertise that cannot be easily transferred to other members. They are designed to carry the intelligence and information found in the intellect of experts and provide this knowledge to other members of the organization for problem-solving purposes.
Typically, the problems to be solved are of the sort that would normally be tackled by a medical or other professional. Real experts in the problem domain (which will typically be very narrow, for instance "diagnosing skin conditions in human teenagers") are asked to provide "rules of thumb" on how they evaluate the problems, either explicitly with the aid of experienced systems developers, or sometimes implicitly, by getting such experts to evaluate test cases and using computer programs to examine the test data and (in a strictly limited manner) derive rules from that. Generally, expert systems are used for problems for which there is no single "correct" solution which can be encoded in a conventional algorithm — one would not write an expert system to find shortest paths through graphs, or sort data, as there are simply easier ways to do these tasks.
Simple systems use simple true/false logic to evaluate data. More sophisticated systems are capable of performing at least some evaluation, taking into account real-world uncertainties, using such methods as fuzzy logic. Such sophistication is difficult to develop and still highly imperfect.
Expert Systems Shells or Inference EngineA shell is a complete development environment for building and maintaining knowledge-based applications. It provides a step-by-step methodology, and ideally a user-friendly interface such as a graphical interface, for a knowledge engineer that allows the domain experts themselves to be directly involved in structuring and encoding the knowledge. Many commercial shells are available, one example being eGanges which aims to remove the need for a knowledge engineer.
Article Source